n. A form of machine learning-based artificial intelligence that enables human operators and researchers to understand how the system derives its results.
2017
The Explainable AI (XAI) program aims to create a suite of machine learning techniques that:
- Produce more explainable models, while maintaining a high level of learning performance (prediction accuracy); and
- Enable human users to understand, appropriately trust, and effectively manage the emerging generation of artificially intelligent partners.
2017
Armed with XAI, your digital assistant might be able to tell you it picked a certain driving route because it knows you like back roads, or that it suggested a word change so that the tone of your email would be friendlier.
2016
The target of XAI is an end user who depends on decisions, recommendations, or actions produced by an AI system, and therefore needs to understand the rationale for the system’s decisions.
2004 (earliest)
The Explainable AI (XAI) works during the afteraction review phase to extract key events and decision points from the playback log and allow the NPC AI controlled soldiers to explain their behavior in response to the questions selected from the XAI menu.
Machine learning (ML) is a subset of artificial intelligence that enables a system to make decisions based not on preset rules, but by using an algorithm to feed huge amounts of raw data through an artificial neural network (ANN)—essentially, a computational model inspired by the workings of animal brains. ML is iterative, meaning that it makes a tentative conclusion, feeds that result back into the ANN (which, being brain-like, changes to reflect the new information), and then repeats until the result has a high probability of being correct. The system has then "learned" what it set out to find, all without being programming explicitly to do so.
The problem is that sometimes ML-based systems—and especially a subset of ML called deep learning that uses multi-layered (hence, "deep") artificial neural networks—come up with results that surprise even the researchers who created the system and programmed its learning algorithm. That's no big deal if the ML system is just trying to recognize cat pictures, but it's huge when dealing with the AIs that underpin near-future technologies such as self-driving cars, automated medical diagnosis, and autonomous miltary drones. How can we trust and manage these systems if they make decisions that are completely opaque to us?
That's the impetus behind XAI, which seeks a new approach to machine learning that creates decision-making systems that can articulate how and why they made those decisions. Will XAI help us understand some of Amazon's more bizarre product recommendations, or why Facebook suggests you celebrate that day five years ago when your dog died? Unlikely, but we can dream.
Source: DARPA
The problem is that sometimes ML-based systems—and especially a subset of ML called deep learning that uses multi-layered (hence, "deep") artificial neural networks—come up with results that surprise even the researchers who created the system and programmed its learning algorithm. That's no big deal if the ML system is just trying to recognize cat pictures, but it's huge when dealing with the AIs that underpin near-future technologies such as self-driving cars, automated medical diagnosis, and autonomous miltary drones. How can we trust and manage these systems if they make decisions that are completely opaque to us?
That's the impetus behind XAI, which seeks a new approach to machine learning that creates decision-making systems that can articulate how and why they made those decisions. Will XAI help us understand some of Amazon's more bizarre product recommendations, or why Facebook suggests you celebrate that day five years ago when your dog died? Unlikely, but we can dream.
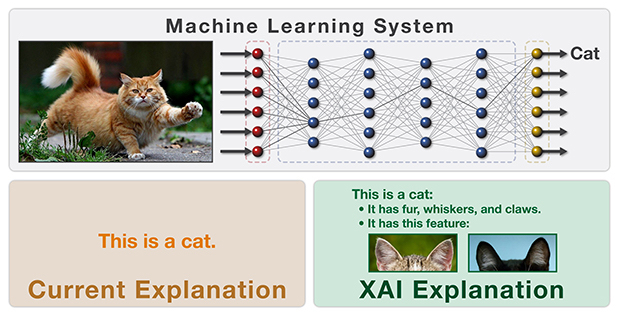